It's still all about the data ✅
To harness the full potential of generative AI, enterprises must start investing in their own data curation infrastructure now.
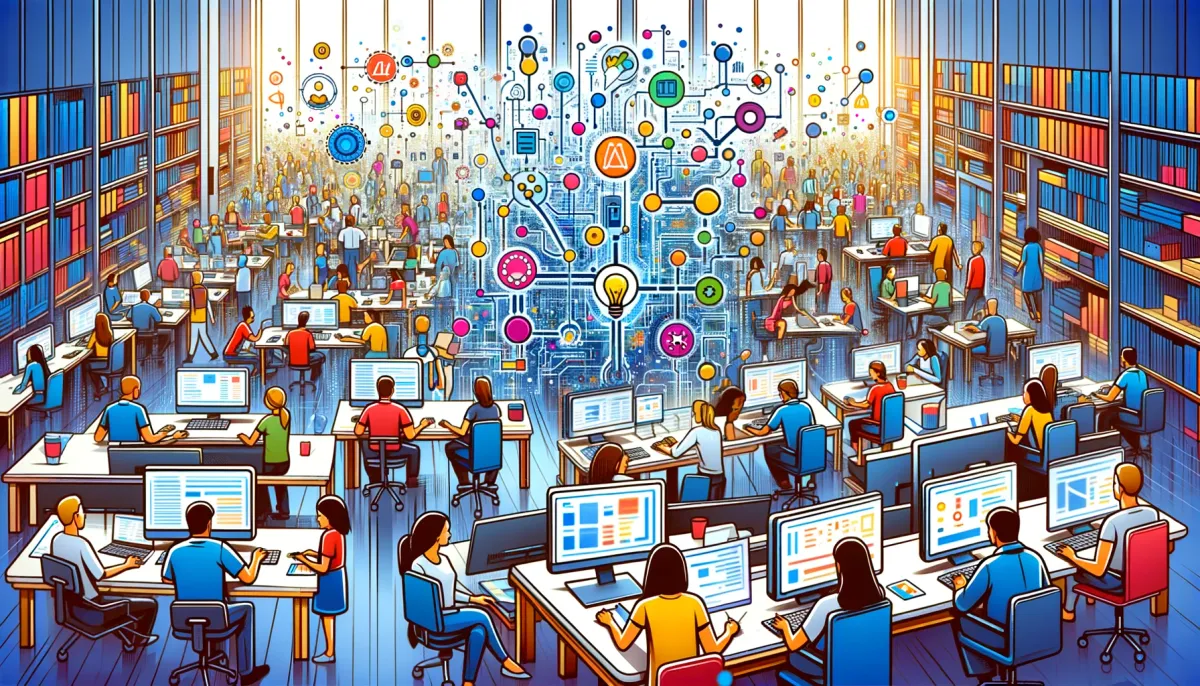
Accessible and easy-to-use large language model (LLM) APIs have made it simple to create impressive generative AI proof of concepts. However, for these AI systems to move from concept to production, they must be validated using high-quality, human-labeled data. This is the sticking point that typically kills an exciting new AI use case, particularly in industries with strict regulatory requirements, where the accuracy and reliability of AI outputs are paramount.
While the exact data used to train models like GPT-4 remains a trade secret, it's clear that the leading AI companies are investing tens of millions of dollars in generating human-labeled data to feed into these models. This investment underscores the importance of high-quality data in the development of robust, reliable AI systems.
To harness the full potential of generative AI, enterprises must start investing in their own data curation infrastructure now. By curating datasets specific to their LLM-based use cases, companies can ensure that their AI models are evaluated using relevant, high-quality data. This investment will not only ensure the accuracy and reliability of AI systems but it will also enable enterprises to fine-tune their own models, reducing their dependence on the big AI companies.
Fine-tuning models using company curated datasets offers several advantages. First, it allows for the development of LLMs that are tailored to the unique needs and challenges of each enterprise. This customization can lead to more accurate and relevant outputs, as the models are trained on data that directly reflects the company's domain and use cases. Second, fine-tuning models in-house gives enterprises greater control over their AI systems, ensuring that they can adapt quickly to changing business requirements and regulatory landscapes.
Just like traditional data science and machine learning projects, it's essential that company leaders recognise the critical role that data plays in the success of generative AI projects. While LLMs have made it easier to explore new AI use cases, the real value lies in the ability to validate and refine these models using high-quality, human-labeled data.
To stay ahead of the curve, data science leaders must advocate for investment in data curation infrastructure within their organisations. This investment should focus on creating datasets specific to the company's LLM-based use cases, allowing AI systems to be validated and enabling the development of fine-tuned models that are tailored to the enterprise's unique needs.
By prioritizing data curation and investing in the development of company-specific datasets, enterprises can unlock the full potential of generative AI, creating more accurate, reliable, and robust AI systems that drive real business value. As the AI landscape continues to evolve, those who recognize the importance of data and invest accordingly will be well-positioned to succeed in the era of generative AI.
Euan Wielewski is an AI & machine learning leader with deep expertise of deploying AI solutions in enterprise environments. Euan has a PhD from the University of Oxford and leads the Applied AI team at NatWest Group.
Want to chat to Euan about AI? Click the button below to arrange a call!