Data Scientist 2.0 🧑🔬
With the advent of generative AI and LLMs, the role of the data scientist is evolving.
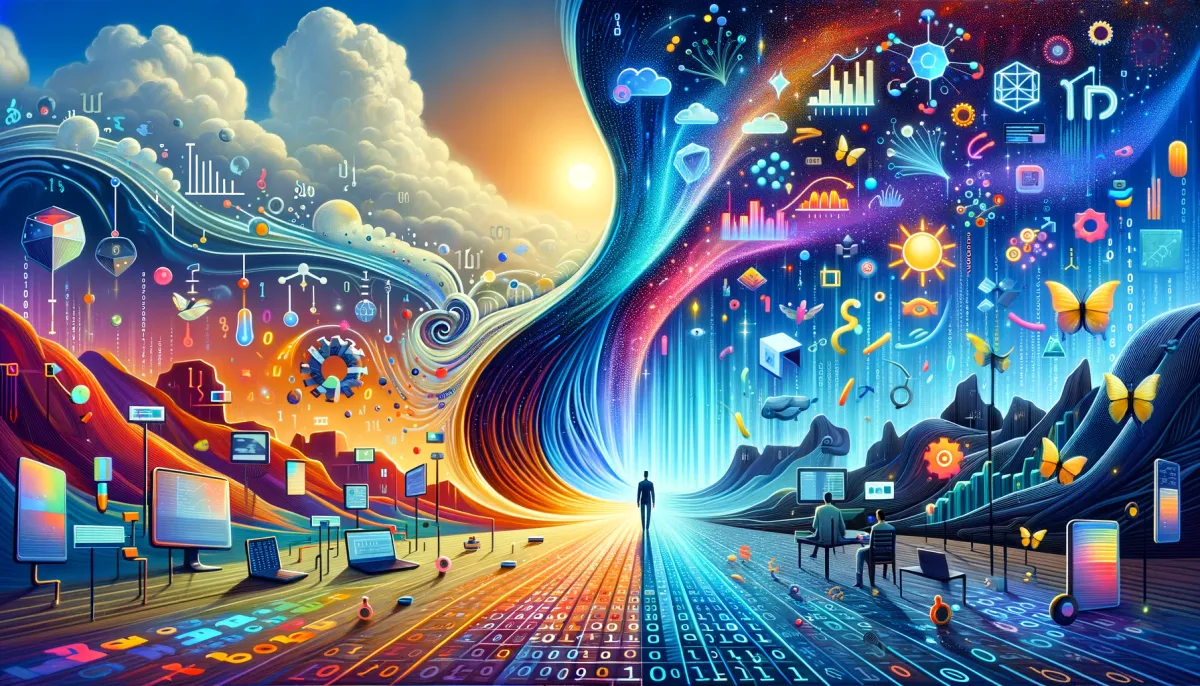
Generative AI and large language models (LLMs) are not only reshaping the way we approach AI projects but also fundamentally altering the skills requirements and team dynamics necessary for success.
Traditionally, data scientists have been the driving force behind AI projects, focusing their efforts on designing and training complex models to extract insights from vast amounts of data. However, with the advent of generative AI and LLMs, the role of the data scientist is evolving. Increasingly, the emphasis is shifting from model development to the evaluation and refinement of outputs generated by these powerful pre-trained models.
This shift has significant implications for data scientists. Many who have thrived on the intellectual challenge of crafting intricate models from scratch suddenly find themselves prompt engineering and evaluating LLM outputs for accuracy and bias. Prompt engineering, while crucial for eliciting desired responses from LLMs, can feel more like an art than a science, requiring a different set of skills and mindset compared to traditional model development.
Moreover, the rise of generative AI is highlighting the need for stronger collaboration between data scientists, software engineers, and subject matter experts. While data scientists bring their expertise in statistical analysis and machine learning, software engineers play a critical role in integrating LLMs into production systems, ensuring scalability, and managing the infrastructure required to support these resource-intensive models. Subject matter experts, on the other hand, provide the domain knowledge necessary to evaluate the quality and relevance of the generated outputs in the context of specific use cases.
To successfully navigate this new landscape, organizations must foster a culture of cross-functional collaboration and continuous learning. Data science leaders must recognize the evolving skill requirements and provide their teams with the necessary training and resources to adapt. This may involve upskilling data scientists in software engineering principles, exposing them to the latest advancements in generative AI, and encouraging them to work more closely with software engineers and subject matter experts.
Furthermore, it is crucial to acknowledge and address the potential impact on job satisfaction among data scientists. Leaders must find ways to keep their teams engaged and motivated, perhaps by emphasizing the strategic importance of their role in evaluating and refining the outputs of generative AI models. Encouraging data scientists to explore novel applications of these models and providing opportunities for them to contribute to cutting-edge research can also help maintain a sense of intellectual stimulation and growth.
In conclusion, the advent of generative AI and LLMs is transforming the skills requirements and team dynamics for AI projects in large enterprise organizations. Data scientists, while still essential, are seeing their roles evolve from model design and training to the evaluation of LLM outputs. This shift necessitates a greater emphasis on cross-functional collaboration between data scientists, software engineers, and subject matter experts. To successfully navigate this new landscape, organizations must foster a culture of continuous learning, provide the necessary training and resources, and find ways to keep data scientists engaged and motivated. By embracing these changes and adapting accordingly, we can harness the immense potential of generative AI to drive innovation and create value for our organizations.
Euan Wielewski is an AI & machine learning leader with deep expertise of deploying AI solutions in enterprise environments. Euan has a PhD from the University of Oxford and currently works at NatWest Group.
Want to chat to Euan about AI? Click the button below to arrange a call!